BryteFlow Data Replication
BryteFlow for SAP BryteFlow for Oracle BryteFlow for SQL Server BryteFlow for PostgreSQL BryteFlow for MySQLReplication Platforms
Amazon S3 Amazon Redshift Snowflake SQL Server Azure Synapse Analytics ADLS Gen2 Apache Kafka Google BigQuery PostgreSQL Databricks Teradata SingleStoreData Reconciliation
What is Data Reconciliation?
The process of data integration starts with replicating data from different sources before it is merged and transformed into a format that is suitable for use on the destination database or system. But before that you need to verify that the target data is the same as in the source system. Data Reconciliation is the term given to this verification of the target data against the original source data.
Why Data Reconciliation is essential
You cannot trust your data without data verification
So you have your data in your Data Lake or Data Warehouse. But how do you know that it is complete, and that there is no missing data? Without high quality, complete data all your clever analytics and data insights simply cannot be trusted. Incorrect data will lead to flawed insights, and that isn’t what you want for your data management projects. How to Manage Data Quality (The Case for DQM)
Full extracts vs Change Data Capture
Some organisations rely on full extracts from the source to prevent data loss. These are cumbersome, take lots of time to extract and load and tax the system heavily. Because of this, full extracts of data are done infrequently, e.g. at the end of the day. Change Data Capture using transaction logs is a much better design pattern for replicating data to the target, as it can be done more frequently. It has zero impact on source and is quick to extract and load. With Change Data Capture, data reconciliation is essential to make sure all the data has landed safely in the destination. Zero-ETL, New Kid on the Block?
Comparing record counts does not always work
You need to constantly verify your data and make sure that some network issue or other infrastructure issue has not prevented the data from being extracted, transformed or loaded into the target. Some organisations rely on record counts and compare source and destination counts. This is better than doing nothing, but it still does not solve the problem completely. If updates are not properly captured or applied, the record counts may be the same but the data can be drastically different.
How to verify data completeness
Data reconciliation has to be done at the column level for the most important columns and this is a tall task for large data sources as it puts a huge load on the source systems and needs a lot of engineering work – an expensive exercise in both respects. And when data is changing and being updated constantly, if the source system does not really have a quiet time or has a very small window for data verification, this is impossible to achieve. Successful Data Ingestion (What You Need to Know)
Untrustworthy data means delays in getting to insight or worse – flawed insights
When your business loses trust in the data, they will try to navigate around the data platform that was built to solve this very requirement – to provide a scalable, trustworthy foundation for all data management projects and insights. Desperate measures equal bad and unusable data. For maximum effectiveness, data reconcilation should be done at record count levels AND at the individual column level with high performance. If there are any discrepancies, the data verification software should provide timely notifications when data discrepancies are found and easy ways to fix these. How to Manage Data Quality (The Case for DQM)
BryteFlow TruData is BryteFlow’s automated data reconciliation and validation software that checks for completeness and accuracy of your data.
Take a first hand look at our data reconciliation tool. Get in touch with us for a FREE Trial.
Effective Data Reconciliation must have these
3 things in place.
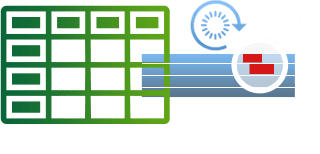
Constant data verification
Source data that is continuously changing and being updated needs to be reconciled constantly so target data in the target destination can be consistent with the source data. Automated data reconciliation is the way to go for data completeness assurance.
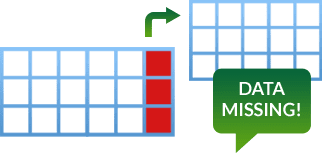
Data reconciliation at record count and column level is ideal
Record counts are not enough to verify data. You need to reconcile data at record count levels as well as individual column levels. Timely notification should also be available in case of missing data.
How to Manage Data Quality (The Case for DQM)
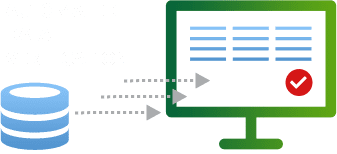
Easy to remedy and easy to use
Data reconciliation should be automated so it is simple to put in place and to remedy situations of imissing data. BryteFlow TruData slices very large tables into easily handled chunks to improve remediation of non-reconciled data, making it easy for you to find and fix the issue.
BryteFlow TruData
BryteFlow TruData is our data reconciliation tool in the BryteFlow suite. BryteFlow TruData verifies your data for completeness constantly so you can be sure no data is missing. BryteFlow TruData uses smart algorithms to reconcile data. It compares row counts and columns checksum in the source and destination data to pinpoint errors.
- 100% data completeness guarantee, you always rest easy!
- Works at a very granular level to reconcile data so it can be remedied easily.
- Integrates with BryteFlow Ingest for data reconciliation automatically.
- Intuitive point and click interface and dashboard for reconciling data.
- Performs point-in-time data completeness checks for complete datasets including type-2.
- Automatic catch-up from network dropout.